PIVOT Courses Associated with Improved SEEQs and Tool Use
Faculty training to support empirically driven course design potentially represents one of the most scalable options available for improving student outcomes at an institution. One strategy to assess effectiveness related to institutionally supported training has been to consider Student Evaluation of Educational Quality (SEEQ) surveys in courses taught by faculty who participated in the training program. For this reason, the UMBC Report Exchange (REX) team has recently released the SEEQs and Other Course Metrics By Training report for the campus community to look further into these data on their own. For those who are unfamiliar with the data warehouse and would like to learn more, please consult this FAQ article to learn how to gain access.
There are two simultaneous advantages of effective training: 1) the better courses are designed, the greater the likelihood unit-level objectives align back to course-level goals and ultimately to institutional functional competencies, and therefore we would expect improved outcomes of various measures, and 2) the better enterprise tools are leveraged through intentional design, the more meaningful the signal – metadata – from activity within a course, and in turn, the more precise predictive modeling might be to inform behavioral nudging.
Similar to prior explorations, this current analysis shows PIVOT courses in terms of average SEEQs and student behaviors in courses as “signal” (i.e., tool interactions). Notably, this trend held when looking at individual instructors’ pre/post training. Overall, there is a statistically-significant SEEQ gain for courses instructed by a PIVOT participant (.09; p<.001), as illustrated above in Figure 1.
Figure 1: Mean Distribution of Bb Course SEEQs, by Term and PIVOT Participation

The difference in averages is even more pronounced when looking at the Fall 2020 and Spring 2021 terms separately, where we see an initial gain of approximately .06 in the Fall, followed by an even greater increase to .11 by the Spring. One way to interpret this increase is the faculty who participated in training began applying new techniques learned through PIVOT in the Fall and then were able to more fully apply these approaches as they honed their pedagogy in the Spring term.
Other treatments, including the Alternate Delivery Program (ADP), which informed PIVOT, as well as ongoing programming in the form of one-time training and webinars, do not appear to have the same positive, measurable impact on SEEQs. Including SEEQs in a model with PIVOT, ADP, and myUMBC training event data shows no significant relationship with SEEQs and either ADP or myUMBC-documented training. In other words, for non-PIVOT trainings, there’s not a similar discernable lift.
PIVOT and ADP courses, on the other hand, tend to also have higher DFW rates (an uncontrolled mean difference of 2% and 2.2%, respectively). However, analysis to address the role of course design using propensity score matching indicates the relationship between training and DFWs is not statistically significant and therefore the increased prevalence of this negative outcome appears to be a function of course design rather than training (i.e., how tools are used, rather than the actual treatment).
Although the methodologies do not control for instructor-level effects, like how one teaches or interacts with students, since both PIVOT and non-PIVOT trained faculty were exposed equally to the pandemic discontinuity – i.e., as a natural experiment there was simultaneous disruption – subsequent mean SEEQ differences are accurate measurements of treatment effects. In other words, maybe the training helped, or maybe PIVOT instructors, on average, contemporaneously and inexplicably earned higher mean SEEQ values due to another yet-to-be-determined variable. However, in the notable absence of such a separate, identifiable catalyst, the mean differences are attributable to the training and the relationship appears causal. Moving forward, DoIT plans to continue investigating PIVOT’s potential impacts on students' learning and engagement as well as faculty satisfaction and course design.
~ by Tom Penniston
There are two simultaneous advantages of effective training: 1) the better courses are designed, the greater the likelihood unit-level objectives align back to course-level goals and ultimately to institutional functional competencies, and therefore we would expect improved outcomes of various measures, and 2) the better enterprise tools are leveraged through intentional design, the more meaningful the signal – metadata – from activity within a course, and in turn, the more precise predictive modeling might be to inform behavioral nudging.
Similar to prior explorations, this current analysis shows PIVOT courses in terms of average SEEQs and student behaviors in courses as “signal” (i.e., tool interactions). Notably, this trend held when looking at individual instructors’ pre/post training. Overall, there is a statistically-significant SEEQ gain for courses instructed by a PIVOT participant (.09; p<.001), as illustrated above in Figure 1.
Figure 1: Mean Distribution of Bb Course SEEQs, by Term and PIVOT Participation

The difference in averages is even more pronounced when looking at the Fall 2020 and Spring 2021 terms separately, where we see an initial gain of approximately .06 in the Fall, followed by an even greater increase to .11 by the Spring. One way to interpret this increase is the faculty who participated in training began applying new techniques learned through PIVOT in the Fall and then were able to more fully apply these approaches as they honed their pedagogy in the Spring term.
Other treatments, including the Alternate Delivery Program (ADP), which informed PIVOT, as well as ongoing programming in the form of one-time training and webinars, do not appear to have the same positive, measurable impact on SEEQs. Including SEEQs in a model with PIVOT, ADP, and myUMBC training event data shows no significant relationship with SEEQs and either ADP or myUMBC-documented training. In other words, for non-PIVOT trainings, there’s not a similar discernable lift.
PIVOT and ADP courses, on the other hand, tend to also have higher DFW rates (an uncontrolled mean difference of 2% and 2.2%, respectively). However, analysis to address the role of course design using propensity score matching indicates the relationship between training and DFWs is not statistically significant and therefore the increased prevalence of this negative outcome appears to be a function of course design rather than training (i.e., how tools are used, rather than the actual treatment).
Although the methodologies do not control for instructor-level effects, like how one teaches or interacts with students, since both PIVOT and non-PIVOT trained faculty were exposed equally to the pandemic discontinuity – i.e., as a natural experiment there was simultaneous disruption – subsequent mean SEEQ differences are accurate measurements of treatment effects. In other words, maybe the training helped, or maybe PIVOT instructors, on average, contemporaneously and inexplicably earned higher mean SEEQ values due to another yet-to-be-determined variable. However, in the notable absence of such a separate, identifiable catalyst, the mean differences are attributable to the training and the relationship appears causal. Moving forward, DoIT plans to continue investigating PIVOT’s potential impacts on students' learning and engagement as well as faculty satisfaction and course design.
~ by Tom Penniston
Posted: August 19, 2021, 3:58 PM
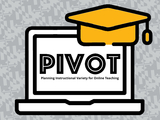