Bb Predict and FYIs Inform Behavioral Nudges
Over the past three full semester terms, UMBC has implemented a predictive analytics pilot “nudge” campaign using Blackboard (Bb) Predict. Beginning in the Spring 2018 term, we selected high enrollment (N ≥ 100) gateway courses based on their student use of the LMS (interactions), strong Civitas signal courses, where a low grade is associated with lower graduation rates, and high rates of students receiving a first year intervention (FYI) and/or D, F or W (DFW).
PSYC100 was identified for a two-tiered messaging campaign informed by students' Bb Predict values. DoIT sent Nudge A, signed by the section’s instructor, Eileen O’Brien, through the myUMBC campus portal to students who had a 50% or greater chance of receiving a DFW and but did not receive an FYI from their instructor. Nudge B was sent to students using the same criteria as Nudge A, but who also received an FYI. Both messages were deployed mid-semester to 1) maximize the validity of the Bb Predict model and 2) allow time to leverage predictions for this intervention while supporting student self-efficacy.
Prior results indicate that both Bb Predict and FYI operating in tandem appear to improve the precision of intervention efforts and complements our well established FYI system by identifying at-risk students who may not have been pinpointed solely through the current FYI system. The pilot has expanded, more than doubling each term, with 107 total interventions across 14 distinct course sections by the end of the Spring 2019 semester. The full list of participating courses and instructors is available here.
Overall, the FYI and Bb Predict processes working together are very accurate and have incorrectly identified less than 1% of students as likely to receive a DFW when they did not (i.e., false positives). If a student is identified by both systems there is an exceptionally high likelihood of their receiving a DFW in the given term. This prediction gives us an enhanced ability to support students through tutoring or advising.
A larger scale rollout could help increase the number of cases to perform a more robust assessment. Additional faculty participation would help us to sharpen our predictions and support more students. We are moving very conservatively in regards to sharing the results of our predictive model with either students or faculty members until we have a much better understanding of how that might impact effort or relationships. The next phase of our research will incorporate additional data into the model that will help us move the prediction window as early in the term as viably possible.
PSYC100 was identified for a two-tiered messaging campaign informed by students' Bb Predict values. DoIT sent Nudge A, signed by the section’s instructor, Eileen O’Brien, through the myUMBC campus portal to students who had a 50% or greater chance of receiving a DFW and but did not receive an FYI from their instructor. Nudge B was sent to students using the same criteria as Nudge A, but who also received an FYI. Both messages were deployed mid-semester to 1) maximize the validity of the Bb Predict model and 2) allow time to leverage predictions for this intervention while supporting student self-efficacy.
Prior results indicate that both Bb Predict and FYI operating in tandem appear to improve the precision of intervention efforts and complements our well established FYI system by identifying at-risk students who may not have been pinpointed solely through the current FYI system. The pilot has expanded, more than doubling each term, with 107 total interventions across 14 distinct course sections by the end of the Spring 2019 semester. The full list of participating courses and instructors is available here.
Overall, the FYI and Bb Predict processes working together are very accurate and have incorrectly identified less than 1% of students as likely to receive a DFW when they did not (i.e., false positives). If a student is identified by both systems there is an exceptionally high likelihood of their receiving a DFW in the given term. This prediction gives us an enhanced ability to support students through tutoring or advising.
A larger scale rollout could help increase the number of cases to perform a more robust assessment. Additional faculty participation would help us to sharpen our predictions and support more students. We are moving very conservatively in regards to sharing the results of our predictive model with either students or faculty members until we have a much better understanding of how that might impact effort or relationships. The next phase of our research will incorporate additional data into the model that will help us move the prediction window as early in the term as viably possible.
Posted: September 25, 2019, 3:11 PM
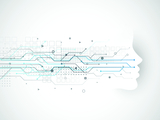